Asian Journal of Control
Special Issue on Optimizing Control in Unmanned Aerial Vehicles Using Evolving Fuzzy Systems and Reinforcement Learning
Guest Editors
Dr. Eduardo P. de Aguiar, Federal University of Juiz de Fora, Brazil
Dr. Saiful Bahri Bin Mohamed, Universiti Sultan Zainal Abidin (UniSZA), Malaysia
Dr. Solomon Adelowo Adepoju, Federal University of Technology, Nigeria
Scope
The brand-new adaptable reinforcement learning control technique uses intermittent ambiguous artificial neural networks to enhance remote aerial vehicles' inclination towards optimizing control performance under challenging flying circumstances. The suggested reinforcement learning processor simply depends on quantifiable environmental information and is therefore not reliant on the functioning of the system. The proposed reinforcement learning technique is used in conjunction with a receptive robustness controller to greatly increase the controller's resilience. Being an inherent approximator, fuzzy systems can express complicated relationships across quantities in the format of fuzzy rules, which is why they are so ubiquitous. Recent technological developments have increased interest in using intelligent unmanned aerial vehicles (UAVs) in both the defence and public sectors. Nevertheless, most fuzzy systems lack dynamic structure, rely on batch learning techniques, and are unable to adjust to quickly changing surroundings. Model-free, data-driven approaches based on developing intelligent systems may be a wise choice, as systems can adjust their settings and design to deal with unforeseen changes in real-time flying behavior.
Reinforcement learning offers many benefits across other approaches, such as the ability to solve complex, dimensional issues that are beyond the scope of standard techniques. the ability to correct errors made throughout development, the ability to provide exceptionally well adaptive learning with respect to typical methods, the ability to function in changing and ambiguous circumstances, the ability to collect data even when it is restricted by the representative's retaliation, and the ability to design greater intelligence control frameworks than those made with traditional methods. It is clear that smart electronics will be required for these sophisticated systems that will equip vehicles for future uses. Self-driving systems have garnered significant interest from academics and professional communities lately. Unmanned aerial vehicles are helpful in many areas, including environmental evaluation, logistics, oversight, and relief efforts. Conservation of energy is one of the main issues with the UAV system that may be solved by applying clustering techniques. Furthermore, deep learning (DL) models must be used for the classification of exceptionally high-frequency remote sensing pictures in order to facilitate efficient decision-making. At present, unmanned aerial vehicles have garnered significant attention from numerous developers and are utilised in several applications, including data aggregation, tracking, and mobility relays.
The special issue highlights the various facets, purposes, and difficulties associated with autonomy. Four possible paths that are seen to be prospective for fuzzy-logic-based methods are compared and analysed. Every time the UAV veers off the planned course, this model forecasts the findings in terms of the weather and the locations of the adversaries. a multidimensional path planning method based on deep reinforcement learning that uses distance metrics and local information instead of worldwide data. In the real world, UAVs with limited sensor capabilities can gather restricted environmental data nearby.
The topics of interest include but are not limited to the following:
• Design of an unmanned aerial vehicle unpredictable actuator utilising reinforced learning.
• Reinforcement learning-based monitoring control optimisation for unmanned aerial vehicle platforms.
• General developing independent neuro-fuzzy control of unmanned aerial vehicles with biological inspiration.
• Assessment: Towards fuzzy reasoning systems in radial wing unmanned aerial vehicles.
• Unmanned aerial vehicle trajectories tracing using fuzzy swarm control.
• Innovative methods and control schemes for self-governing rotorcraft unmanned aerial vehicles.
• Developing accessible intelligent systems for unmanned aerial vehicles using rule-driven technique.
• A fuzzy algorithm system with learning capabilities for controlling unmanned aerial vehicles.
• Unmanned vehicle dynamic scheduling utilising fuzzy logic and optimisation control.
• Ideal fuzzy logic control without models for sovereign unmanned aerial vehicles.
• Optimal horizontal rotation rehabilitation for unmanned aerial vehicle using reinforcement learning.
Important Dates
Deadline for Submissions: August 5, 2024
Completion of First Review: October 31, 2024
Completion of Second Review: January 31, 2025
Receipt of Final Manuscript: February 25, 2025
Publication Date: May, 2025 (Tentatively Vol. 27, No. 3)
Please click here or check the attachment for more details regarding to the special issue!
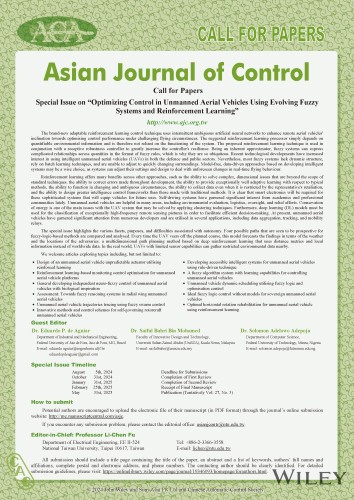
Feel free to contact us at asianjcontr@ntu.edu.tw if you have any question.
Please submit your manuscript at https://mc.manuscriptcentral.com/asjc !